Featured
Baskin Engineering professor reveals flaws in widely-used machine learning method
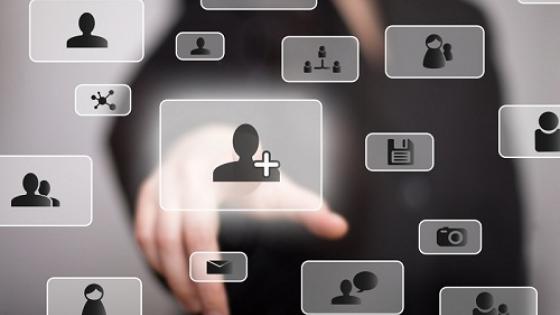
By Amy Baxter
AI in Healthcare
March 26, 2020 — Santa Cruz, CA
(Image source: AI in Healthcare)
UCSC Professor C. “Sesh” Seshadri explains shortcomings in low dimension embeddings
One algorithmic approach used to model and analyze complex networks is fundamentally flawed and fails to capture the full properties of real-world complexities, according to a new study published in Proceedings of the National Academy of Sciences.
Specifically, the techniques known as low-dimensional embeddings, which are used as input to machine learning models, have shortcomings despite being commonly used.
“It’s not that these techniques are giving you absolute garbage. They probably have some information in them, but not as much information as many people believe,” C. “Sesh” Seshadhri, first author of the study and associate professor of computer science and engineering in the Baskin School of Engineering at UC Santa Cruz, said in a statement.
One example of how the method is flawed is within social networks, which is a complex network that many companies want to use machine learning in order to predict social behavior and recommendations for users. A person’s position in a social network is converted into a set of coordinates in a geometric space through embedding techniques, which then creates a numbered list of people used in an algorithm. The conversion allows the system to make predictions based on the relationships in the points in space, according to the press release.
Continue reading here: https://www.aiin.healthcare/topics/research/widely-used-machine-learning-method-flawed
###